Auxetic materials with the counter-intuitive effect of negative Poisson’s ratio (NPR) have potentials for diverse applications. Typical shape optimization designs of auxetic structures involve complicated sensitivity analysis and a time-consuming iterative process, which is not beneficial for designing functionally-graded structures where the auxetics at different locations need to be inversely designed. To improve the efficiency of the inverse design and simplify the sensitivity analysis, we propose a deep-learning-based inverse shape design approach for tetra-chiral auxetics. First, a non-uniform rational basis spline (NURBS)-based parameterization of tetra-chiral structures is developed to create design samples and computational homogenization based on isogeometric analysis is used in these samples to generate a database consisting of mechanical properties and geometric parameters. Then, the database is utilized to train deep neural networks (DNN) to generate a surrogate model that represents the effective mechanical properties as a function of geometric parameters. Finally, the surrogate model is directly used in the inverse design framework where sensitivity analysis can be calculated analytically. Numerical examples with verifications are presented to demonstrate the efficiency and accuracy of the proposed design methodology.
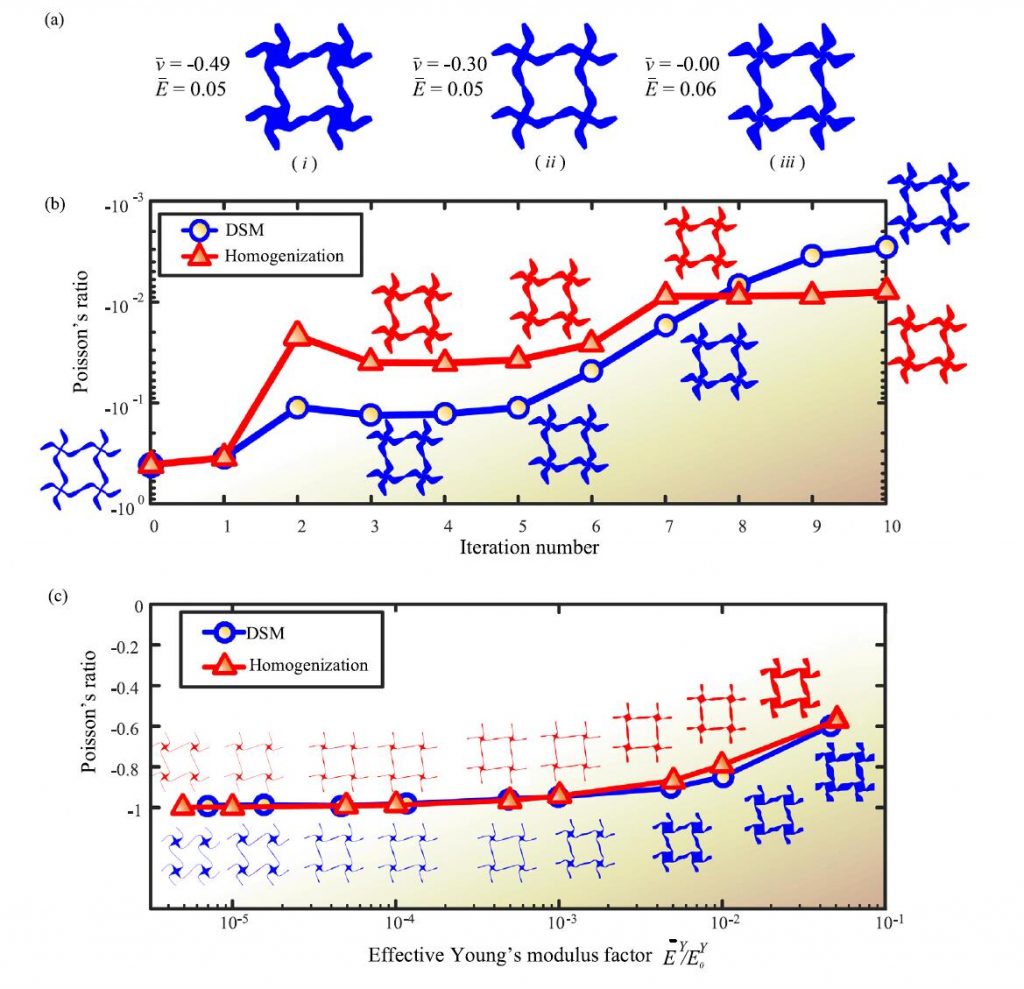